I was so excited to see the announcement of the M6 forecasting competition. For those who don’t know the M series of competitions (not to be confused with BMW cars) is a long running series of competitions for forecasting time series. It comes from the 1980’s, well predating the modern data science explosion. And this M6 is probably the worst of the entire series. It is a flawed attempt to predict the entire stock market.
I have been hoping to get my forecasting package, AutoTS, into a good competition, and I just missed out on the M5 competition. For the M5, AutoTS wasn’t really ready yet. I also didn’t have a proper workstation, and my laptop wasn’t up to running the not-yet-optimized AutoTS setup, so I didn’t have the horsepower to give it a proper go. A few years latter, both problems had been fixed and I’m ready for a contest.
Let’s first look at the good things about the M6 competition. The M6 competition is live – meaning you forecast stuff before it actually happens and are later evaluated. The M5 was historic data, people willing to put in the effort could have dug up the historic promotions, weather, and so on, and then made sure their model went into an appropriate direction, cheating (the winners didn’t, but it was a risk).
The biggest problem with the M5 competition was it was only on a single evaluation sample. Forecasting is never perfect, the winner of a particular evaluation sample is always going to have some element of luck involved. The winner of the M5 even acknowledged that on the forum – they had a similar model to other top people, and just got lucky that theirs was the closest. The M6 fixes that. There are now 12 evaluation windows, each for one month of a year. The overall winner is going to be overwhelmingly determined by skill, with a smaller role for luck.
Now let us start with one huge problem: the M6 competition is about forecasting the stock market. Okay, I acknowledge that this is where the money is. The cash prizes are big, and I can only bet that some big hedge fund or bank is looking to crowdsource a bit of knowledge. But the stock market is insane. Everything that happens on the planet ties into it. Natural disaster? War? Plague? Consumer sentiment of billions of people? Some CEO having an affair and getting fired? There are so many factors, and many of them are impossible to predict. Maybe one market segment would be doable, but this competition is a broad swath of the entire market. The people that will win, if they participate, will be the big companies that have hundreds of analysts researching together to create a clear profile of what they do and don’t know. Little old me, with a few hours on the weekend, no, not possible.
Quite simply, the next competition should be live, and have multiple evaluations, but should be on a mix of smaller-scope data. Some weather, natural data like river flow volume, or solar flares. Cool kids these days think about the environment and I am sure there are plenty of ecologists and astronomers happy to provide a data stream. Other possibilities are some product demand/sales data (which is the most common type of forecasting in industry and was used in the M5), or web traffic data (there’s open source government web traffic data that could be used). More diversity, and ideally things that don’t require predicting the entire global economy and news cycle.
Ideally the data would be weekly – right now with stocks you have just the weekend to submit – from the freshest data ending market-close on Friday to the submission deadline around noon central time on Sundays. Having a full week from fresh data to submission deadline would be nice. Also, it would be nice if the organizer provided data. With the M6, you have to source your own stock data, which increases the barrier to entry and makes contestants, like me, uncertain if I am actually predicting the right thing. I don’t know for sure, but I am willing to bet number of participants for this competition is lower than they were hoping.
Let’s look at some more problems. My biggest complaint overall is actually the ranking metric for forecasting. The ranked probability score (RPS) is niche in general, but especially for the stock market. For this competition, you have to accurately predict which quintile the returns for that stock will be, ranked relative to all other stocks. One problem: I could accurately predict a handful of stocks very accurately, and then make a great investment decision on those. But if I don’t have an accurate forecast for all 100 stocks, my rank will be useless. So the metric is useless if you do quite well on one segment but not on all segments.
Secondly, a basic truth of the stock market is that most stocks will follow the market most of the time. Which means the actual values of returns will generally all be very close. So even if you had a very good forecast saying that most stocks in a group would give around a 6% return next month, your ranked forecast might still be useless because the little 6.01% vs 5.99% differences would determine your ranking but those little differences are essentially random. I have absolutely no clue how someone chose RPS for the forecast metric for the stock market. It is clearly the worst possible choice. Absolute accuracy (good old MAE, SMAPE, etc), not some ranking nonsense, should be used for scoring.
And I should note I have actually tested this. The RPS accuracy of my forecasts has no correlation with any other forecasting metric. And I have tried dozens of ways of trying to combine forecasts into rankings. My best models are around +/- 1.5-2% SMAPE on stock value after one month across cross validation. I consider that phenomenally good given the problems mentioned above. Yet their ranking performance is still essentially random, as caused by the reasons outlined above.
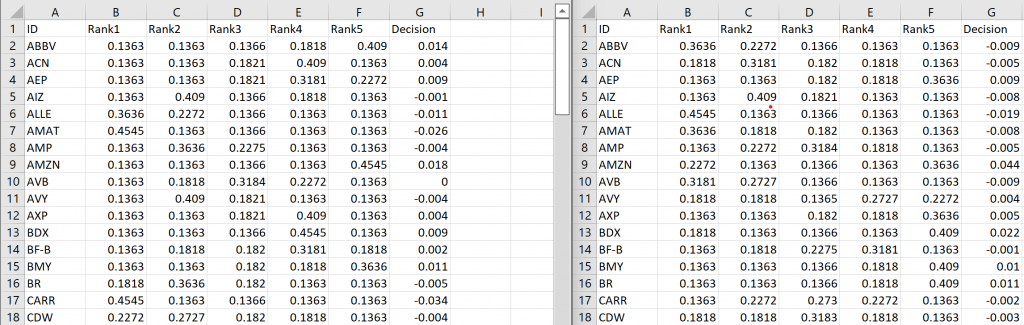
Stock data also presents one minor challenge for the other half of the scoring criteria, the investment decision. Most good stock market forecasts are pretty close to 0 on returns. There is a nearly equal chance of the market going up or down. My ideal solution is to do a full AutoTS run that takes about a day, then repeat that a few times, then manually combine those different runs to see, across multiple runs, are there any stocks consistently showing a strong tendency either up or down, then go all in on those most likely candidates. However, I don’t have enough time in the one weekend of submission time, to do that (I’ve got other hobbies and chores). I could build a system that does that automatically, but geez, building an entire automated investment platform is no simple undertaking.
I think human+machine hybrid is probably the smartest investment strategy for this competition, but doing that every month for 12 months is more time than most people want to spend. Again, this competition clearly favors industry personnel (especially since they sometimes have quasi-insider levels of market knowledge) and doesn’t really favor any sort of technical expertise that is more broadly applicable to other forecasting domains.
In summary, two suggestions for next time: don’t try to predict the entire stock market, and use a better scoring metric. I could be wrong, maybe some cool new algorithms will come out of this competition. But I think most likely it will just show that organizations with massive stock market knowledge machines already built will vastly outperform some bored little guys tinkering on the weekends. We already knew that. I personally invest mostly in a mix of index funds and mutual funds, and see that as unlikely to change no matter how good I get at forecasting otherwise.
You are ranked second in the IR category. Congratulations.
Pingback: Winning the M6 Financial Forecasting Decision Category – Syllepsis